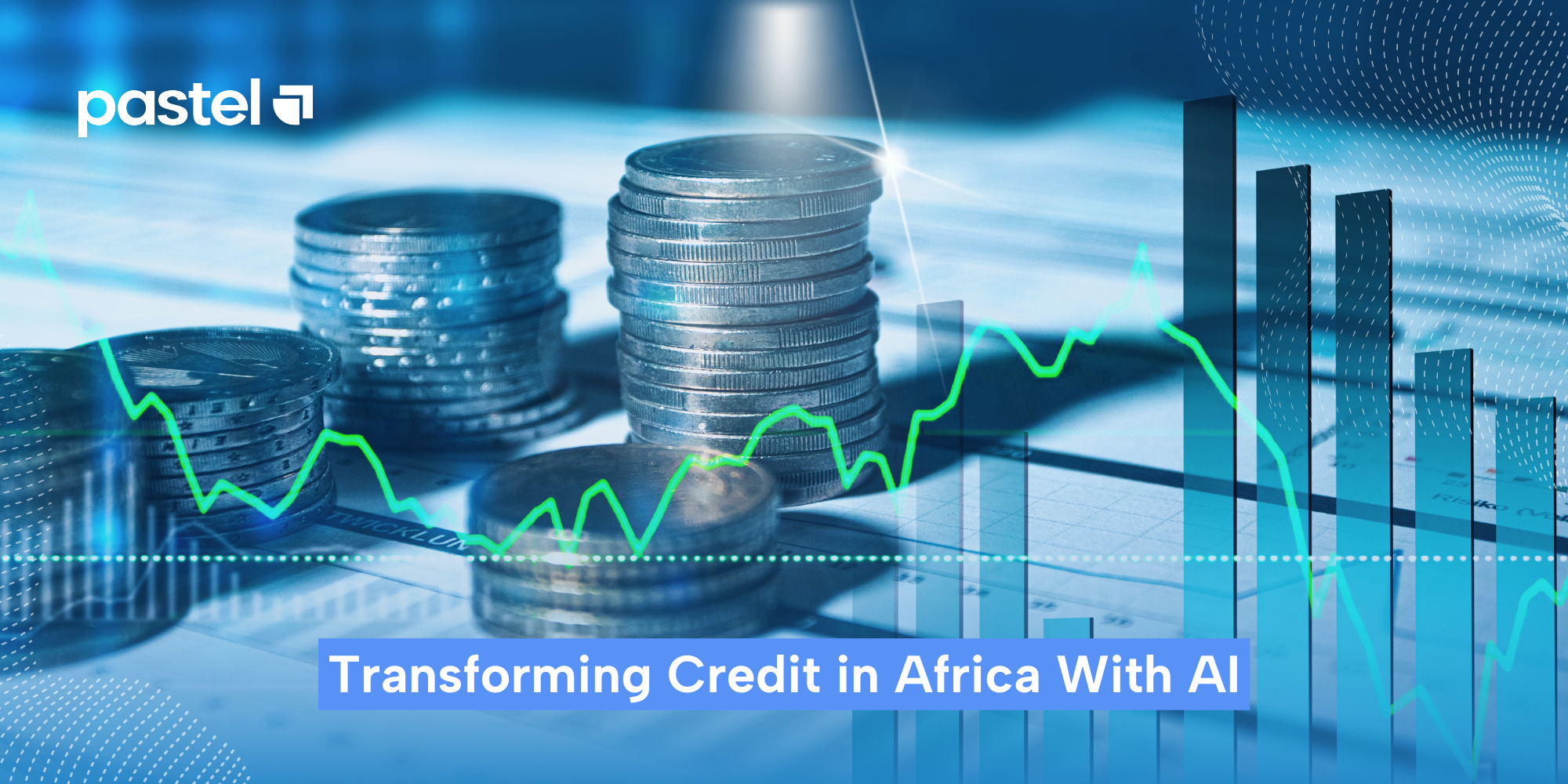
Unlocking Financial Access: Transforming Credit Scoring Systems for the Unbanked in Africa with AI
Introduction: Understanding Financial Access
In emerging economies like Africa, the concept of financial access holds significant importance, as policymakers and stakeholders are increasingly recognizing its potential impact on macro-economic growth. Financial access entails providing individuals and businesses with the opportunity to utilize a range of financial services, including payday loans, cash loans, deposits, insurance, and more. Policymakers see enabling financial access as a crucial avenue for enhancing people's overall well-being, alleviating poverty, and driving economic development. In the context of this article, our focus will be on the role of AI-driven credit scoring systems in paving the way for extensive financial inclusion across Africa. Hang in tight!
The Concept of Credit Scoring Explained
The concept of credit is simply used to determine how financially sound an individual or business is for financial products such as cash loans, payday loans, and more. Practically, it works this way — a creditor extends a loan to a debtor and receives interest as compensation for this service. However, a critical question arises: how can the creditor determine whether the debtor will repay the loan? The creditor faces the potential of financial loss if the debtor fails to honor their repayment commitment. This necessitates the development of a predictive model that assesses the level of risk associated with a debtor's repayment behavior, a concept known as credit risk. To mitigate this risk, creditors often implement strategies such as requesting collateral or adjusting interest rates based on the borrower's assessed level of risk, a practice referred to as risk-based pricing.
Credit scoring is an analysis performed by a lender or financial institution to determine the credit worthiness of an individual or a business in need of a loan. The process of credit scoring basically involves the attribution of a credit score to an individual or business which helps lenders and financial institutions determine whether such a person or business qualifies for financial products such as payday loans, cash loans, mortgages, and credit cards. The process of then confirming the qualification of such creditworthiness is referred to as credit check.
The Approach to Credit Scoring
The global recession of 2008 played a pivotal role in driving the development of standard credit scoring systems. The main reason for the recession was tied to borrowers not being able to pay back their loans. Lenders advanced loans to people with poor credit and a high risk of default. A surge in mortgage approvals led to an upswing in demand for homes, consequently driving up housing prices. As a result, these homeowners used their properties as collateral to secure substantial loans. However, when faced with repayment obligations, many found themselves unable to fulfill them. This predicament proved to be problematic for banks as the value of the homes fell short of covering the outstanding debt. The repercussions were substantial, and led several banks to declare bankruptcy due to the overwhelming magnitude of these losses.
These challenges then led to the introduction of Basel II Accord, a set of international banking regulations released by the Basel Committee on Banking Regulation which was formed to help countries standardize their rules and alleviate market anxiety regarding risks in the banking system.
As a reaction to the global financial crisis of 2008, the Basel II Accord was propounded on three main pillars: minimum capital requirements, regulatory supervision, and market discipline.
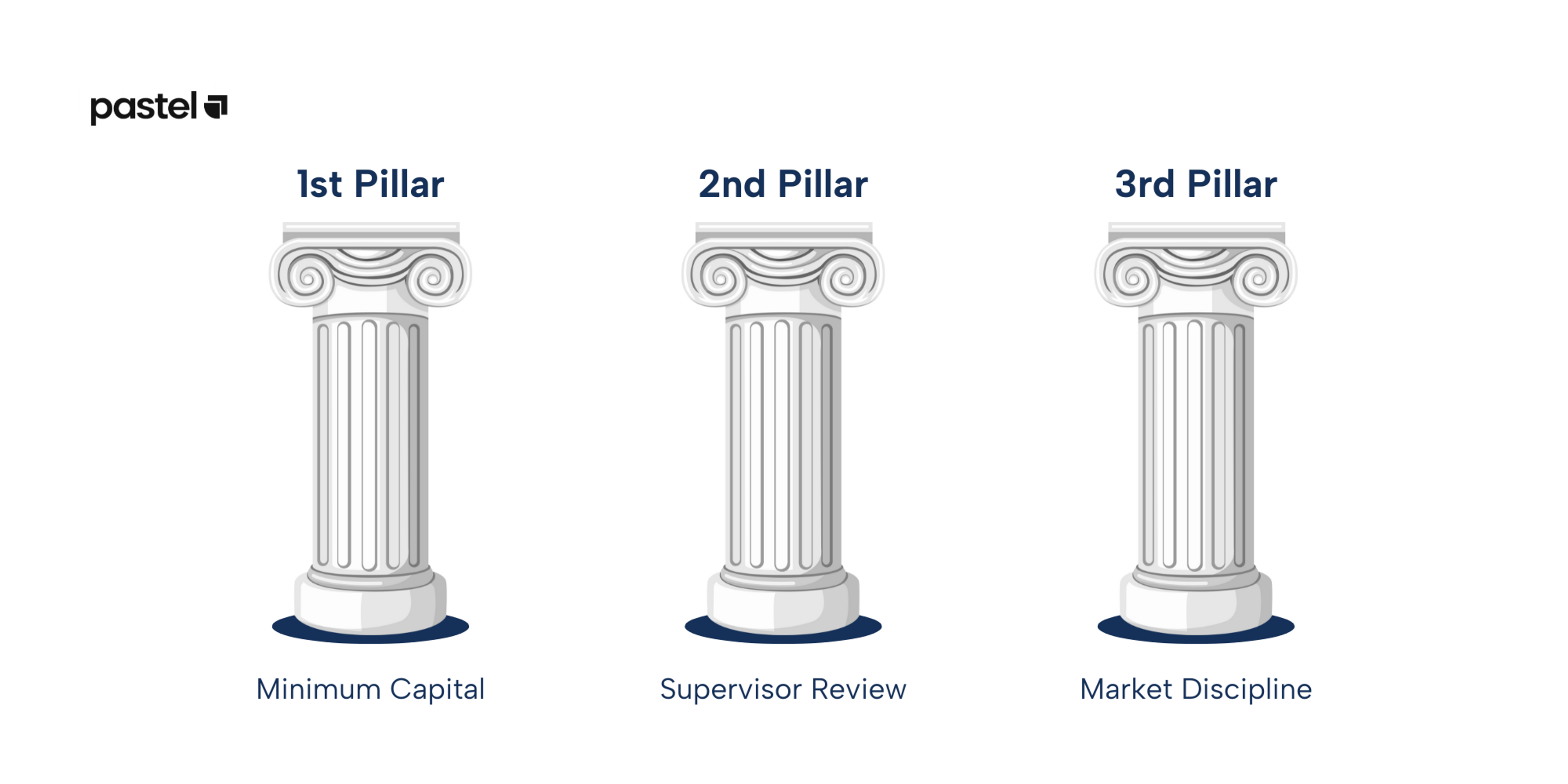
The approach to credit scoring hinges on the first pillar, requiring that banks maintain a capital reserve equivalent to a minimum of 8% of their risk-weighted assets. This signifies that risk estimation has evolved from an optional practice to a regulatory imperative for banks. They are now required to predict the potential loss associated with each credit given out, fundamentally being the sum a borrower might default on.
A primary formula for calculating Expected Loss (EL) is:
EL = PD x LGD x EAD
Here's a breakdown of the components:
- PD (Probability of default) signifies the probability that a borrower will be unable to fully or promptly repay their debt.
- LGD (Loss given default) denotes the percentage of the asset that's irretrievably lost when a borrower defaults.
- EAD (Exposure at default) represents the total value that a lender is at risk of losing when a borrower defaults.
For instance, let's consider this scenario:
A borrower secures a $40,000 loan but defaults after repaying $10,000, with records showing that 30% of borrowers in that area default. The borrower also used a car valued at $20,000 as collateral.
Calculation:
EAD = $40,000 - $10,000 = $30,000 - $20,000 = $10,000
PD = 30%
LGD = $10,000 / $30,000 = 33.33% or 1/3
Expected Loss
Basel II Approaches to Credit Risk
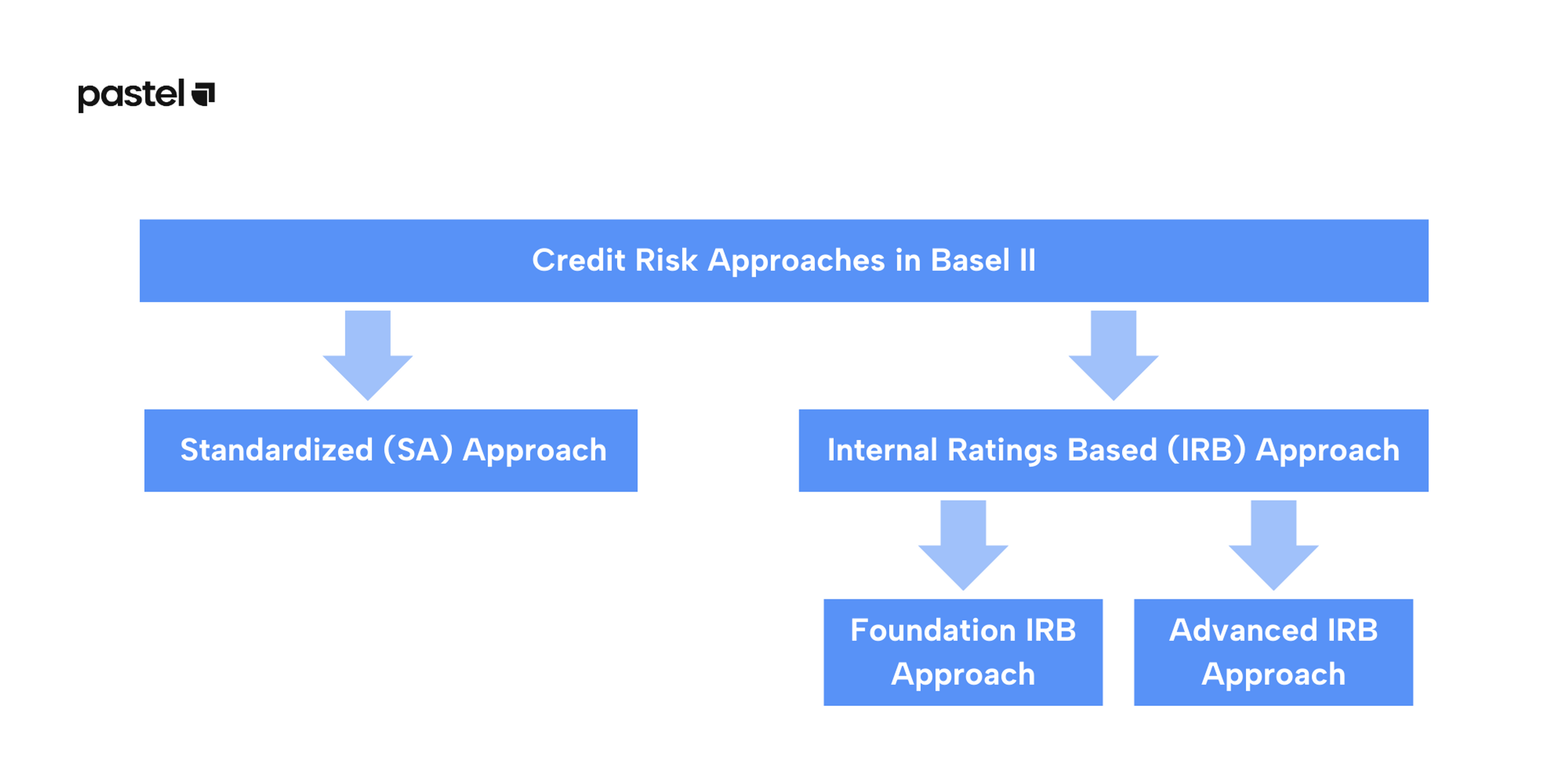
Under the Basel II regulation, banks can assess credit risk (EAD, PD and LGD) in two different ways, namely:
• The Standardized (SA) Approach
• Internal Ratings-Based (IRB) Approach
The standardized approach to credit scoring includes using an external accredited agency, such as FICO, to assess an individual's creditworthiness for a loan. FICO score is one of the prominent external scores commonly used for this purpose. The higher an individual's FICO score, the stronger their creditworthiness; conversely, a lower score indicates weaker creditworthiness. This methodology was predominantly approved by regulators during the initial implementation phase of Basel II in numerous countries as part of their alignment with the requirement to estimate credit risk through external credit rating agencies.
In contrast, the Internal Ratings-Based (IRB) Approach comprises two key components:
1. The Foundation IRB approach involves the internal estimation of the PD (probability of default) for borrowers. This allows financial institutions to assess the likelihood that a borrower might default on their loan obligation using their own internal data and models.
2. The advanced IRB approach takes the internal estimation a step further. Under this approach, financial institutions are allowed to internally estimate not only the PD (probability of default), but also the LGD (Loss given default) and EAD (Exposure at default) components. This comprehensive approach enables financial institutions to have a more subtle understanding of the risk profile associated with their lending to a borrower in any given situation.
What Does Credit Scoring in Africa Look Like?
Currently, the credit rating system in Africa is primarily dominated by global credit rating agencies like Moody’s, S&P, and Fitch, which collectively control around 95% of the credit rating market worldwide. These agencies assess the creditworthiness of various entities, including individuals, and corporates, aiding investors in gauging risk and potential returns. However, amid this landscape, it is worth highlighting the diversity of credit assessment practices across the continent.
There are lots of credit bureaus across Africa, each with their methods and ways of assessing and modeling credit risk. While these local credit bureaus play a significant role in providing credit information, their approaches can differ significantly from those employed by international credit rating agencies. This diversity underscores the complexity of the credit rating landscape in Africa, where local factors, market nuances, and regional dynamics come into play. In light of this, it's clear that a more localized and tailored approach to credit scoring is essential to address the unique challenges faced by the African market.
It’s important to note, however, that with these credit rating agencies come many credit biases and inconsistencies as their credit systems are not based on the African context. Concerns have arisen due to these agencies' swift downgrades of individuals and businesses who default and their slow upgrades for African nations. There has also been the concern of inadequate risk perception assessment, limited stakeholder consultation, and questions about their impartiality. A recent UN study revealed that these subjective biases have collectively cost African countries a staggering US$74.5 billion which have impacted funding opportunities and led to higher interest payments on public debt. Given these challenges, there's a growing momentum towards establishing a localized credit rating mechanism for the continent. Such an approach could ensure more contextualized, reliable credit assessments, addressing Africa's unique economic landscape and fostering financial inclusion.
The Way Forward: Transforming Credit Scoring Systems in Africa
Transforming the credit scoring system in Africa holds immense significance, as it offers the potential to provide financial access to the more than 50% of the population that currently remains unbanked on the continent. The adoption of artificial intelligence models presents a pivotal opportunity for realizing this transformation, with its potential to mitigate credit biases and streamline credit risk assessment.
Among a range of possible methods aimed at creating AI-driven credit scoring models, two distinct approaches prominently come to the forefront. The first is the straightforward linear model scorecard approach, which begins with comprehensive data exploration and cleaning. At this stage, features are engineered based on the specific market dynamics. In this approach, the resulting dataset is then transformed using the Weight of Evidence (WOE) method by transforming all independent variables based on the proportions of defaulters and non-defaulters to discriminate between good and bad loan applications.
Continuous variables are then split into bins, calculating the percentage of good defaulters and bad defaulters to estimate WOE values, while for categorical values, the binning step is omitted. Information value (IV) of each variable is then measured for each variable and only variables that have high IV are selected. This value measures the predictive power of each variable. Logistic regression is subsequently applied to the transformed dataset resulting in a credit scorecard created by model coefficients and WOE scores.
In contrast, the deep learning approach takes a different trajectory. Beginning with data exploration, cleansing, and feature engineering, this method leverages a non-linear model to predict the probability of credit loan default. The predicted probability output undergoes scaling and other transformation to provide a credit score. What sets this approach apart is that the model itself is used as a tool for credit score prediction.
By adopting either of these approaches, African banks and financial institutions can navigate loan approval decisions with increased efficiency and impartiality. At Pastel, our commitment to this transformation is evident in our dedicated team's expertise, the existing datasets we have access to, and our strategic partnerships. With the convergence of these factors, we are uniquely positioned to contribute significantly to ushering in a new era for Africa's financial landscape, driven by the power of artificial intelligence.
In future blog posts, we will further explore the application of deep learning models for credit assessment in Africa. Stay tuned!